Is Your Business Really Ready for AI?
AI has become a powerful tool for companies looking to stay competitive. But here’s the catch: jumping into AI without proper preparation can do more harm than good.
Many businesses are eager to adopt AI, but enthusiasm alone doesn’t guarantee success. In fact, a large number of AI projects fail—not because the technology isn’t good, but because the organization simply isn’t ready. Whether it’s messy data, unclear goals, or unrealistic expectations, the most common problems with AI have nothing to do with the tech itself.
So how do you know if your company is truly AI-ready?
In this article, we’ll walk through five red flags that signal your business may not be prepared for AI implementation—yet. More importantly, we’ll show you how to fix each one, so you can move forward with confidence.
At Sunmait, we work with companies at every stage of AI adoption—offering support from strategic planning to full-scale implementation. If you're unsure where to start, we’re here to help.
Why AI Fails in Business (Before It Even Starts)
AI promises efficiency, automation, and smarter decision-making—but the road to success is rarely smooth. In reality, a significant number of AI initiatives either stall, underperform, or fail entirely. And the reasons usually have little to do with the sophistication of the algorithms or the quality of the software.
So, what’s going wrong?
In most cases, AI struggles because businesses rush in without the right foundation. According to industry research, up to 70–80% of AI projects fail to deliver the expected results. Why? Because of issues like:
Poor data quality or fragmented systems.
No clearly defined AI use case.
Lack of skilled personnel.
Incompatible legacy infrastructure.
Unrealistic expectations about ROI or timeline.
These are not technical problems—they're organizational problems. Businesses treat AI like a plug-and-play tool, when in fact it requires a careful blend of data readiness, strategic planning, internal skills, and iterative deployment.
This is especially true for medium-sized companies, where resources are often limited and every tech investment needs to justify its cost. Adopting AI without a clear game plan can burn both time and budget—fast.
The good news? You don’t need to be a tech giant to benefit from AI. You just need to be AI-ready—and that starts by knowing what warning signs to watch for.
In the next section, we’ll dive into the five red flags that might be holding your business back from successful AI adoption—and show you how to address each one.
Red Flag 1: Poor Data Quality or Incomplete Datasets
AI systems thrive on data. Whether you're training a machine learning model or setting up basic automation, the accuracy and completeness of your data directly impacts the success of your AI project. Unfortunately, many businesses underestimate just how problematic their data infrastructure really is.
Signs this is a problem:
Data is scattered across multiple departments or tools (aka data silos).
Duplicates, inconsistencies, or missing values are common.
Your team spends more time cleaning data than analyzing it.
You’re not confident your data is accurate—or even usable.
How to Fix It: Start with a Data Readiness Check
Before diving into AI implementation, conduct a data audit:
Map your data sources: Understand where your data lives—CRM, ERP, spreadsheets, cloud platforms, etc.
Evaluate data quality: Look at accuracy, completeness, consistency, and timeliness.
Break down silos: Use integrations or data platforms to centralize key information.
Set up ongoing data governance: It’s not just about cleaning data once—it’s about maintaining quality over time.
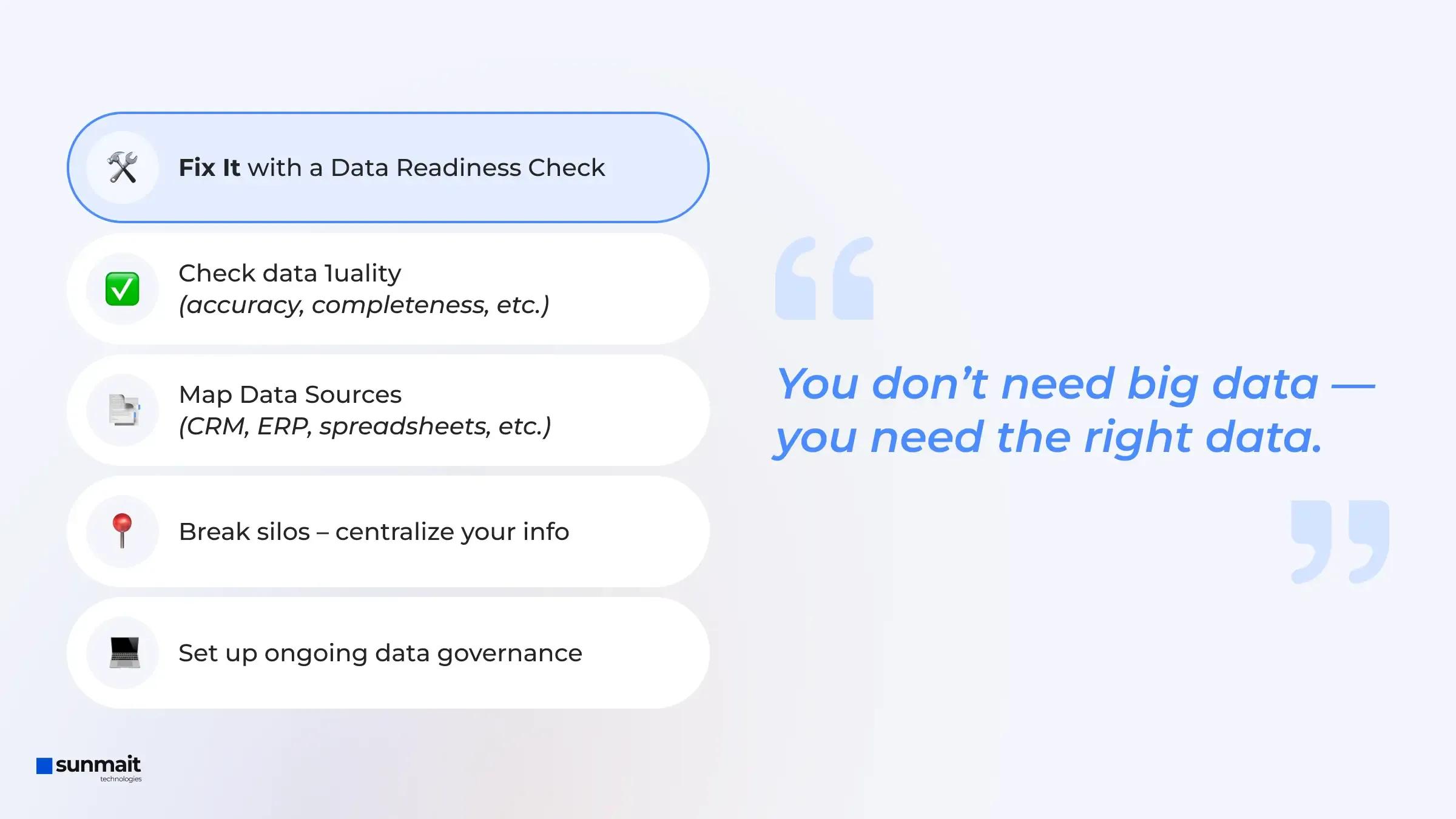
Consider working with a partner who understands both AI systems and business data architecture. At Sunmait, we help companies prepare their data infrastructure to ensure a smooth transition to AI-powered operations.
Remember: You don’t need big data—you need the right data.
Red Flag 2: No Clear Use Case for AI
One of the most common reasons AI initiatives fail is that businesses dive in without a clearly defined purpose. They’ve heard the buzz, they know their competitors are using it, and they want to stay ahead—but they don’t actually know why they’re using AI or what problem it’s meant to solve.
Why this matters:
Without a concrete use case, you risk:
Investing in tools you don’t need.
Chasing vague goals with no measurable outcomes.
Burning time and resources with little to show for it.
AI works best when applied to specific, repeatable, and measurable problems. And in many cases, simpler automation or analytics tools could achieve similar results—without the complexity.
What a clear use case looks like
1. A good AI use case typically includes:
A well-defined problem or opportunity.
Reducing customer churn by predicting when clients are likely to leave.
Automating the processing of incoming job applications or customer service tickets.
Speeding up invoice approvals by auto-extracting and validating key data.
Forecasting product demand to optimize inventory management
2. Available data to support the task.
You should already have access to historical data (e.g., customer interactions, past invoices, application forms, support logs, etc.) that AI can learn from.
3. A measurable business outcome:
Saving 100+ hours per month in manual work.
Reducing response time to customer inquiries by 30%.
Improving forecasting accuracy to reduce overstock by 20%
The more specific and outcome-driven your use case is, the easier it will be to prioritize, implement, and measure success.
Examples of strong AI use cases
To make things more concrete, here are a few realistic, high-impact use cases where AI can deliver measurable value—especially for medium-sized businesses:
Customer retention
Use AI to analyze customer behavior and transaction patterns to predict which customers are at risk of canceling their subscription or not renewing a contract. This allows your team to take proactive steps—like sending special offers or personalized messages—to reduce churn and increase lifetime value.
Document automation in legal or finance
AI can automatically classify, extract, and organize data from contracts, invoices, or loan applications—saving hours of manual work. For example, a legal firm could speed up contract reviews, or a financial team could auto-process incoming invoices for faster approvals.
Application screening in HR
Use AI to analyze job applications and rank candidates based on predefined criteria like experience, keywords, or qualifications. This shortens the recruitment process and frees up your HR team to focus on top candidates faster.
Product recommendation in E-commerce
Train AI models to analyze customer preferences, browsing history, and past purchases to deliver personalized product suggestions in real-time. This leads to better user engagement, higher conversion rates, and increased average order value.
Demand forecasting for inventory management
AI can detect trends and predict future demand based on sales history, seasonality, and external factors. Retailers and manufacturers use this to optimize stock levels, reduce waste, and improve supply chain planning.
Weak use cases:
“We want to be more AI-driven”.
“Let’s add AI to our product because it’s trending”.
How to Fix It: Start with Business Problems, Not Technology
When exploring AI, the biggest mistake companies make is focusing on the tools instead of the outcomes. The real question isn’t “How can we use AI?” — it’s “What’s slowing us down or costing us money that AI could help fix?”
The best starting point is to talk with your internal teams. Sales, support, logistics, and finance often know exactly where inefficiencies are buried. Their insights can reveal repetitive tasks, manual processes, or growing data overload—all areas where AI can make an immediate difference.
Once those challenges are on the table, you can begin shaping them into practical use cases. AI works best when tied to specific business goals and metrics.
Map AI to business goals
Start by identifying the key pain points—like high customer churn, slow service response times, or inefficient manual workflows—and align them with clear outcomes. You might set targets like reducing customer service response times by 40%, automating invoice reviews to speed up approvals, or improving forecasting accuracy to cut down on surplus inventory.
Prioritize low-hanging fruit
Rather than tackling complex, abstract problems, begin with straightforward, repetitive tasks where AI can show value fast. Tasks like invoice processing, application screening, or demand forecasting are ideal. For instance, a retail business might experiment with dynamic pricing to optimize margins based on real-time market signals.
Run a Pilot Before Going All In
Launching a pilot allows you to validate your use case on a smaller scale, learn what works, and fine-tune before investing further. This step builds internal buy-in and minimizes risk.
Leverage Expert Guidance
If your team lacks deep AI expertise, partnering with consultants can dramatically improve your success rate. The right partner can help identify opportunities, assess feasibility, and design solutions tailored to your industry. At Sunmait, we help growing businesses do exactly that—connecting real-world problems with high-impact AI implementations.
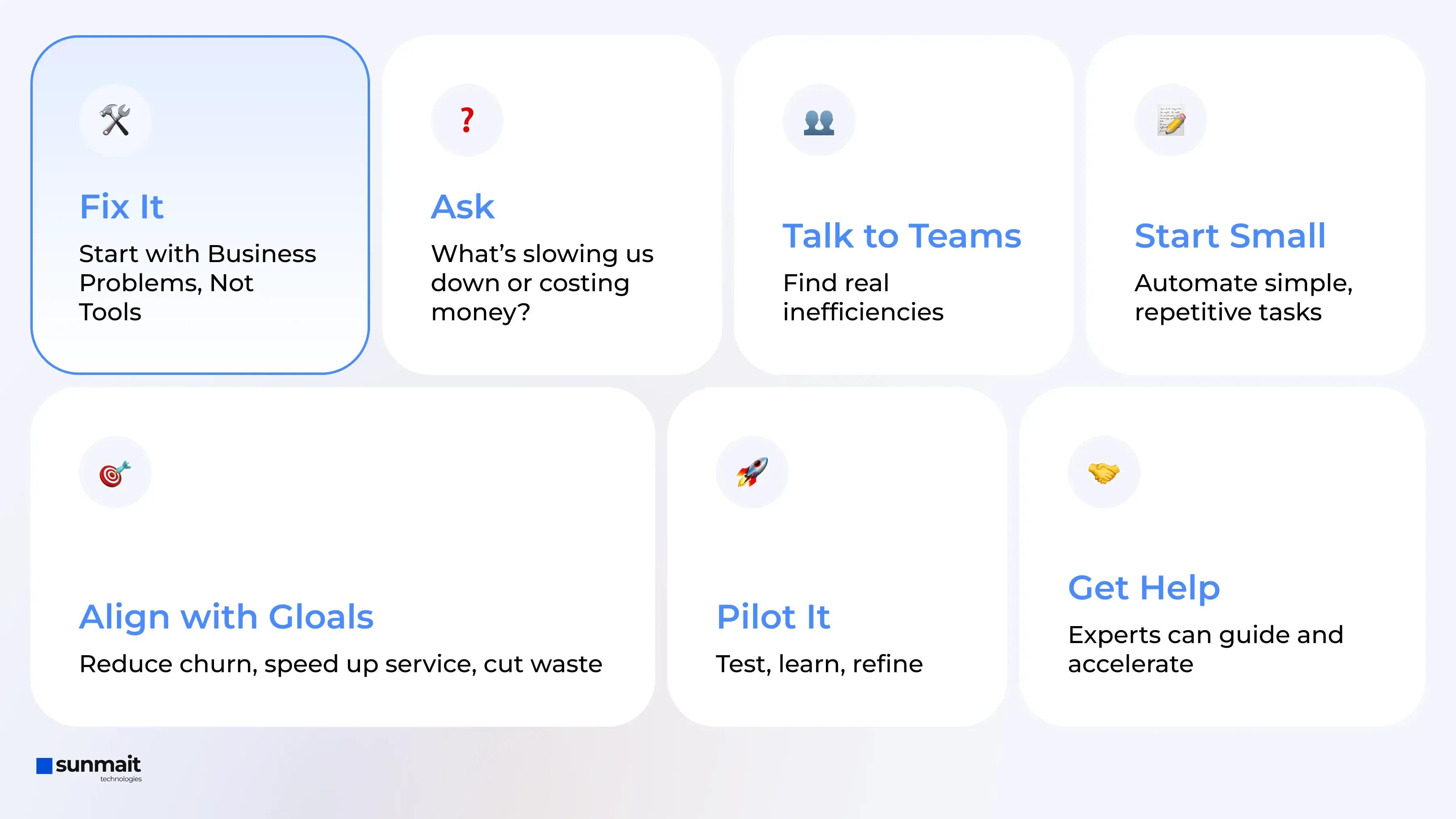
Red Flag 3: Legacy Systems That Can’t Integrate with AI Tools
Even if your business is sitting on years of valuable data and has a clear use case in mind, outdated technology can stop your AI journey in its tracks.
Legacy systems — custom-built platforms, old CRMs, outdated ERPs — often weren’t designed with modern AI tools in mind. As a result, integration becomes a bottleneck, data gets trapped in silos, and AI systems are forced to work with incomplete or incompatible inputs.
Why this is a serious risk:
Legacy systems can quietly derail your AI plans. They may seem stable on the surface, but under the hood, they often create major roadblocks that slow progress, increase costs, and introduce risk. Here’s why:
No modern integrations – Many lack APIs or connectors, making it hard for AI tools to access data.
Manual data extraction – Without automation, getting usable data becomes slow and error-prone.
Bottlenecks in real-time use – AI needs fast, clean data. Legacy systems can’t deliver at the speed AI requires.
Security risks – Outdated platforms often miss critical updates, creating vulnerabilities when connected to new tech.
Hidden costs – Retrofitting AI into old systems drains time, budget, and IT resources.
How to Spot the Problem:
Your core business tools are more than 5 – 10 years old with no recent updates.
Data is only accessible via spreadsheets or manual exports.
Integrating with modern software requires heavy developer involvement.
You've had to build “workarounds” just to get systems to talk to each other.
How to Fix It: Modernize Strategically, Not All at Once
You don’t need to replace your entire tech stack to adopt AI. Start by identifying the core systems AI will rely on — like your CRM, ERP, or databases — and focus on those first.
Begin with a quick audit to spot integration issues, such as missing APIs or incompatible data formats. Prioritize systems tied to critical workflows — like customer data, inventory, or billing — that AI tools need access to.
Modernization tips:
Assess integration feasibility before starting any project.
Prioritize systems tied to key data flows or AI use cases. Use middleware or hybrid solutions to connect old and new tech.
Consider phased cloud migration for flexibility and long-term ROI.
Partner with experts to upgrade smoothly and minimize risk
At Sunmait, we help businesses modernize their infrastructure step by step — ensuring every change supports AI integration while keeping disruption to a minimum.
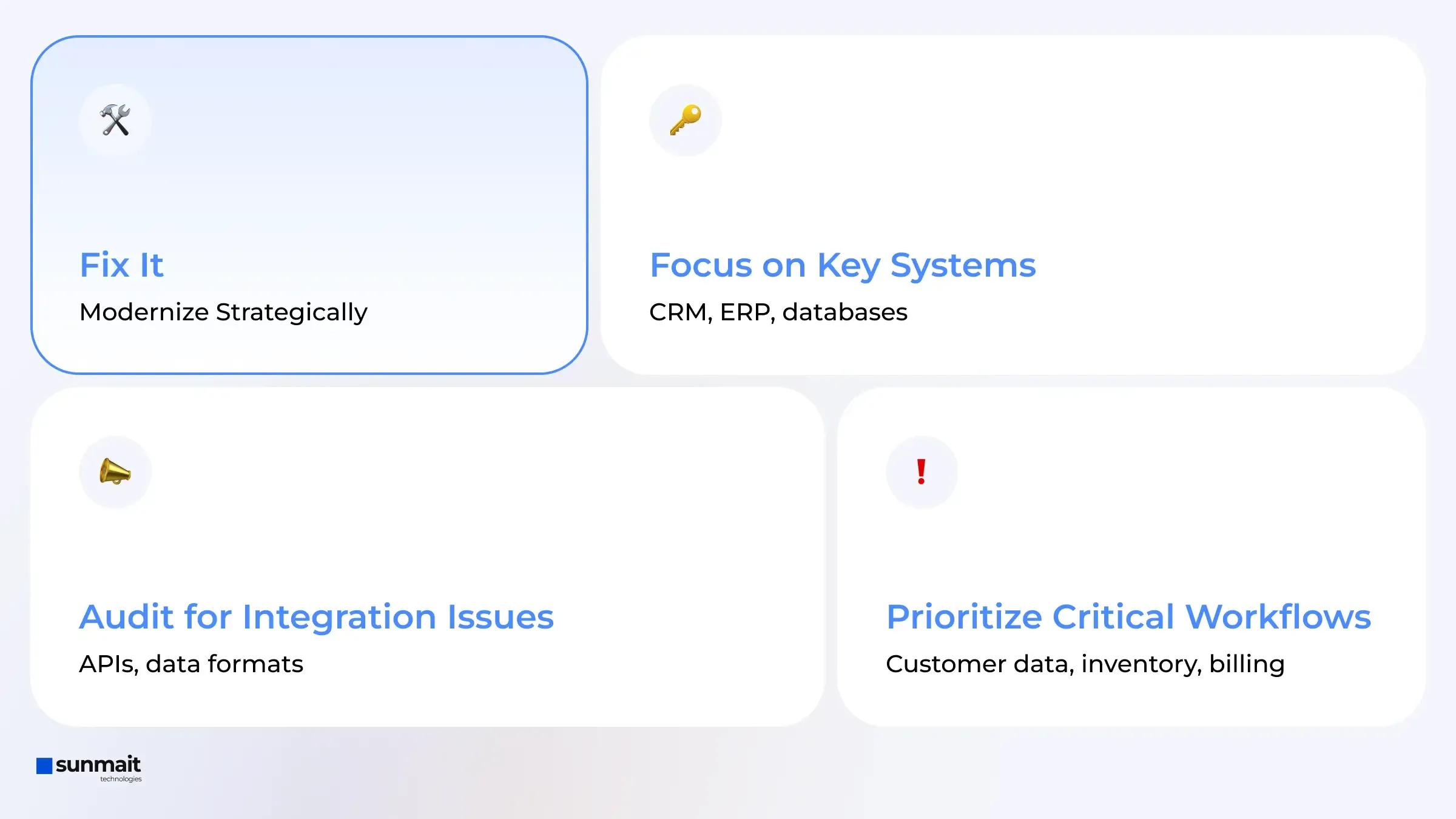
Red Flag 4: Lack of In-House AI Skills
Your AI project can still stall if you don’t have the right people to drive it forward.
AI isn’t just about plugging in software—it involves understanding data science, model training, infrastructure, evaluation, compliance, and more. If your internal team lacks experience with these processes, the learning curve can quickly become a major obstacle.
Signs this might be holding you back:
You rely heavily on third-party tools but don’t fully understand how they work.
No one on your team feels confident explaining AI decisions or outputs.
Projects stall because there’s no internal knowledge to guide or adjust them.
You struggle to evaluate AI vendors, platforms, or service providers.
Off-the-shelf tools can’t be customized to fit your specific workflows.
Your team can’t maintain or update models as data changes.
You're increasingly dependent on external developers, driving up costs and delays
While you don’t need a full data science department, you do need someone who understands the language of AI—and how to align it with business goals.
How to Fix It: Build, Buy, or Partner
You don’t need a full AI department to move forward—you just need the right mix of internal skills, smart hiring, and outside expertise.
Upskill your existing team by investing in targeted training, such as workshops on AI basics, data literacy, or certifications for the tools you plan to use. Focus especially on roles that interact with AI outputs, like analysts, operations managers, or team leads. Encourage cross-functional learning that combines domain expertise with data awareness.
Hire strategically by bringing in hybrid talent—people who understand both business and tech, not just coders. This could be a data analyst or machine learning engineer who can lead small-scale efforts while also aligning with business objectives. For short-term needs, freelance platforms can be a flexible way to access technical support without a long-term commitment.
Partnering with experts when building a full in-house team isn’t practical. Working with AI consultants or implementation partners (like Sunmait 👋) provides immediate know-how while also helping your team build long-term self-sufficiency. You don’t need a PhD to succeed with AI—what matters is having the right support at the right time.
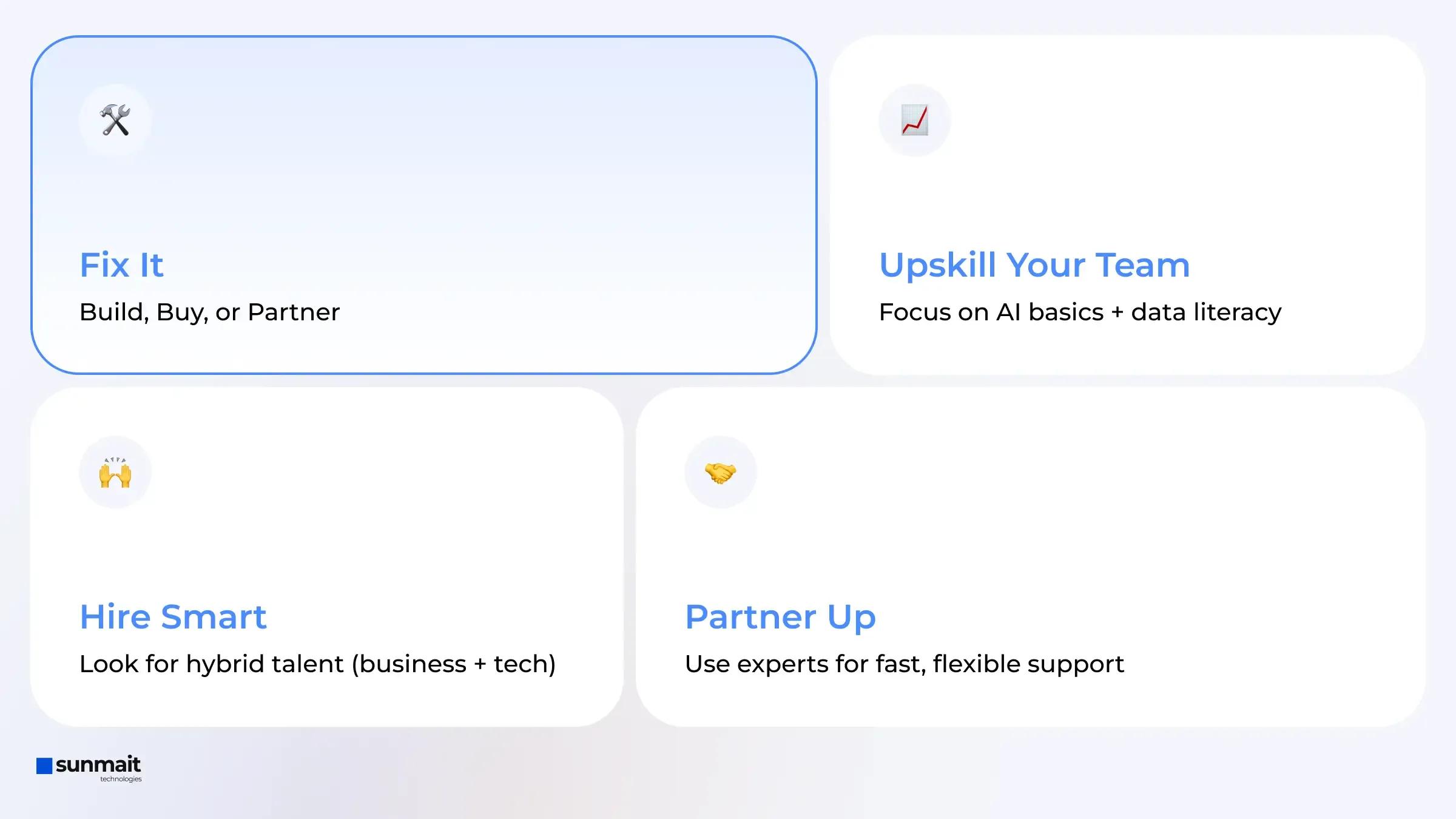
AI success doesn’t require a giant in-house team—but it does require the right expertise at the right time.
Red Flag 5: Unrealistic Expectations About ROI
AI has been hyped as a game-changer—and it is. But for many businesses, especially those just starting out, expectations around return on investment (ROI) can be unrealistically high or dangerously vague.
Leaders often expect fast, dramatic results: instant cost savings, fully automated processes, or predictive insights that change the game overnight. The reality? AI success is typically incremental, and ROI builds over time.
Common misconceptions:
“AI will cut our operational costs in half within months”.
“We’ll automate everything and reduce headcount immediately”.
“Once we implement AI, it runs itself”.
In truth, AI involves experimentation, iteration, and cross-team collaboration. It often starts slow—especially while refining models and cleaning data—but compounds value with time and scale.
How to Fix It: Set the Right Metrics and Mindset
Instead of banking on overnight transformation, treat AI as a long-term capability—not just a one-off project.
Break ROI into short-, mid-, and long-term goals:
Short-term: Improved data visibility, reduced manual workloads, and early efficiency gains.
Mid-term: Measurable improvements in speed, accuracy, or cost reduction.
Long-term: Scalable automation, smarter decision-making, and a stronger competitive edge.
Overpromising — especially from external vendors—can lead to disillusionment and abandoned projects. A phased approach helps manage risk, maintain momentum, and build stakeholder trust as results grow over time.
Measure business impact, not just model performance
Don’t get lost in technical KPIs like model accuracy without tying them to real business outcomes. For example, a customer churn model with 95% accuracy might sound impressive—but if it doesn’t actually help your team retain more customers or reduce cancellations, it’s not delivering meaningful value. Instead, focus on impact-driven results like increased retention rates, reduced support costs, or higher customer lifetime value.
Educate stakeholders early
Set clear expectations internally about the AI timeline, budget, and risks.
Share early wins—even small ones—to build confidence and momentum.
At Sunmait, we work with companies to design realistic AI roadmaps with measurable goals, phased rollouts, and scalable value — not just flashy proof-of-concepts.
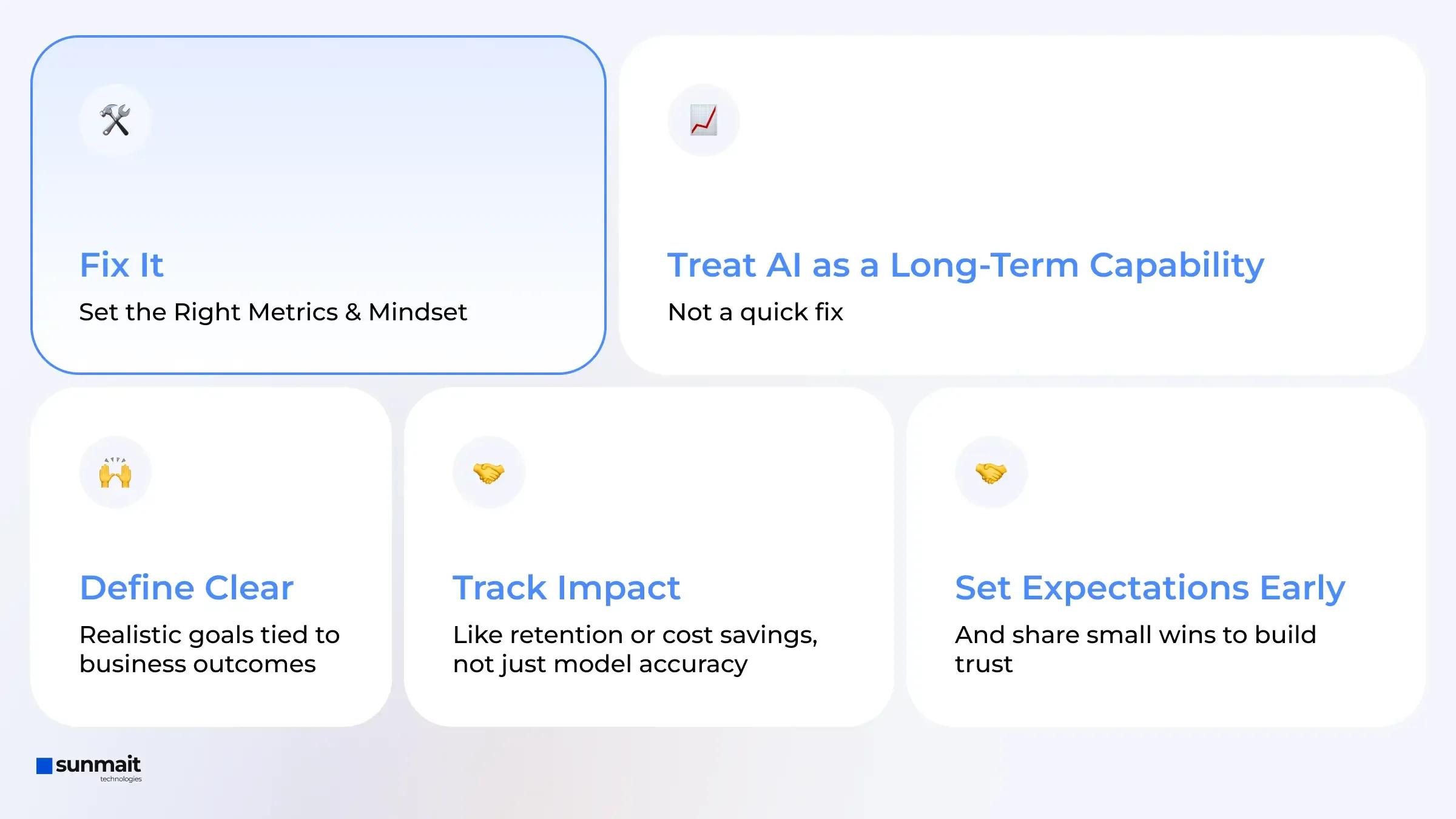
Building AI Readiness: What To Do Next
If you've seen your business reflected in one (or more) of the red flags above, you're not alone—and you're not behind. The truth is, most businesses aren't fully AI-ready when they start exploring the technology. But with the right strategy, tools, and support, readiness isn’t far out of reach.
The key is to see AI as a step-by-step process, not something that works instantly.
Becoming an “AI-powered company” doesn’t happen overnight. It requires a foundation of the right data, clear goals, integration-ready systems, skilled people, and realistic expectations.
Your AI readiness checklist:
We have a clear business problem where AI could make a real impact.
Our data is in good shape—it’s accessible, reliable, and well-organized.
Our current systems can connect with AI tools (or we know how to bridge the gaps).
We have the right people—or trusted partners—who can handle implementation.
Everyone understands that AI takes time, and we’re prepared to roll it out in phases.
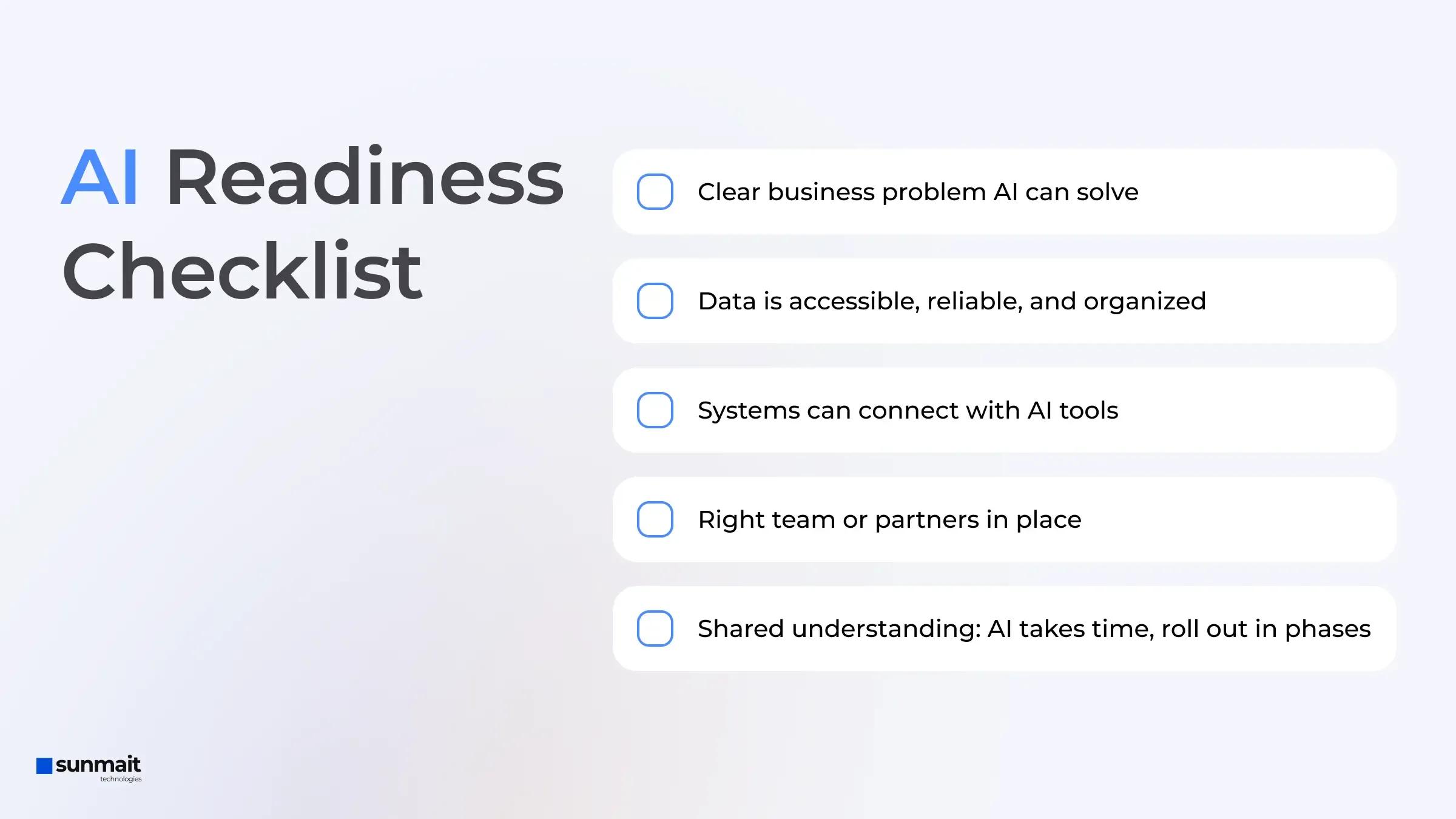
If you can confidently check off most of these boxes, you’re likely ready to move forward with a pilot or proof of concept. If not, that’s okay—the key is knowing where the gaps are so you can fix them before investing heavily.
How Sunmait Can Help
At Sunmait, we help medium-sized businesses bridge the gap between ambition and action. Whether you're starting with a readiness assessment, defining your AI use case, or building a custom AI solution, we work with you every step of the way to ensure your investment pays off—practically and sustainably.
Not sure where to begin? Let’s talk. We’ll help you assess where you stand, where AI can create the most value, and how to move forward — confidently.